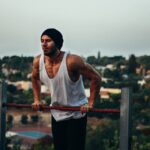
A Beginner’s Primer on Generative AI: Everything You Should Know
January 11, 2024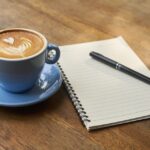
4) Breaking New Ground: The Role of Deep Learning in Generative AI Technologies
January 12, 2024
Hello there, friends! 😊 If you’re as fascinated by artificial intelligence as I am, you’re in for a treat! Today, I want to guide you through an exciting aspect of AI – the choice between Generative AI and Discriminative AI. These two paradigms are like the yin and yang of the AI spectrum, each with its own unique capabilities and applications.
Whether you’re a seasoned AI developer, hobbyist, or just AI-curious, understanding the differences between these two approaches is crucial. So, buckle up as we dive into a comprehensive guide to help you determine which AI model suits your specific needs the best.
But before we begin, let’s set the stage with a friendly reminder: if you enjoy creating mesmerizing images from text descriptions, you’ll love our platform, DrawMyText. Our premium text-to-image generation platform brings your words to life with stunning visuals. Stay tuned to learn more about our exciting features and pricing!
Understanding Generative AI
Generative AI models are a marvel of the AI world. They essentially learn from an assortment of data inputs and understand how to generate new data instances that could pass as ‘real.’ Generative models are known for their ability to create, imagine, and innovate data in various forms, such as images, text, and sounds.
An excellent example of generative models in action is the DALL-E system by OpenAI, which generates images from textual descriptions. So what makes generative models compelling? It’s their capacity to capture the underlying probability distributions of the data they’re trained on. This characteristic enables them to produce new instances that are indistinguishable from actual data.
One aspect of Generative AI that’s important to note is that they’re computationally intensive. They often require significant resources to train and tune. However, once they’re up and running, they can be astonishingly powerful tools for creative tasks, data augmentation, and even predictive modeling.
Deciphering Discriminative AI
On the other side of the coin is Discriminative AI. Discriminative models, or classifiers, excel at identifying which category new inputs belong to based on historical data. If Generative AI is all about creating, Discriminative AI is about recognizing and categorizing.
These models shine when it comes to tasks such as email filtering, disease diagnosis, and language translation. Discriminative models learn the boundary between categories, often producing more accurate predictions for classification and regression problems than their generative counterparts. For a deeper understanding of discriminative models, Google’s glossary on AI terms is a handy resource.
Discriminative AI’s strength lies in its efficiency and accuracy. It typically requires less computational power than Generative AI, making it a go-to choice for many real-world applications where real-time performance is key.
Choosing Between Generative AI and Discriminative AI: A Comprehensive Guide
Choosing between Generative and Discriminative AI models can feel like a daunting task. However, the decision largely hinges on the problem you’re trying to solve. If your objective is to generate new data or discover the underlying structure of datasets, Generative AI is your go-to. Conversely, if you need to classify data or make predictions based on existing labels, Discriminative AI is the way to go.
To make an informed decision, consider the following factors:
- Complexity of the task: Generative models are better suited for complex tasks that require understanding and manipulation of data distributions.
- Availability of data: Discriminative models can work with less data and still perform well, whereas generative models require more data to learn effectively.
- Computational resources: Generative models demand more computing power. Ensure you have the necessary infrastructure before you commit to this approach.
For those interested in the technical depths of Generative vs. Discriminative models, the Stanford AI Lab’s publication on this topic is a treasure trove of information.
Embracing Generative AI with DrawMyText
Generative AI also has incredible applications in the world of art and design, where creativity holds the reins. If this excites you, let’s talk about DrawMyText. Our platform utilizes state-of-the-art Generative AI to turn your textual descriptions into vivid images.
Our offerings cater to various needs, whether you’re an illustrator seeking inspiration or a business looking to create compelling marketing visuals. With competitive pricing and intuitive features, DrawMyText can enhance any project with the magic of AI-driven artistry. I encourage you to check out our pricing and features page and consider subscribing to unlock the full potential of your imagination.
Remember, choosing Generative AI is more than a technical decision; it’s a commitment to innovation and creativity. And with platforms like DrawMyText, you can easily harness its power.
FAQ: Choosing Between Generative AI and Discriminative AI: A Comprehensive Guide
What’s the core difference between Generative AI and Discriminative AI?
The fundamental difference lies in their approaches: Generative AI models learn how to generate new data that is similar to the training set, while Discriminative AI models focus on recognizing and categorizing input data based on learned patterns from the training set.
Can Generative AI models be used for classification tasks?
While Generative AI models are primarily designed for data generation, they can indirectly perform classification by comparing likelihoods. However, Discriminative AI models are usually more efficient and accurate for direct classification tasks.
What are some common applications of Generative AI?
Generative AI is widely used in creative fields for tasks like image and video generation, music composition, text generation, and drug discovery. They are also applied in data augmentation to supplement training data for machine learning models.
Is one type of AI model better than the other?
No, Generative and Discriminative AI models serve different purposes and are better suited for specific tasks. The choice depends on the requirements, goals, and constraints of the problem at hand.
How do I decide which AI model is right for my project?
Assess your project needs, such as the type of task (generation vs. classification), the availability of computational resources, and the quantity and quality of your data. These factors will guide your decision on whether to choose Generative AI or Discriminative AI.
Final Thoughts
In conclusion, whether you’re tilting towards Generative AI or Discriminative AI, the exciting world of artificial intelligence has a lot to offer. Both models come with their strengths and are transformative when applied to the right context. Remember, the best AI model for you is the one that aligns with your specific goals and resources.
And don’t forget, for those of you with a creative streak or a need for high-quality images generated from text, check out DrawMyText – it’s a stroke of generative genius at your fingertips! 🎨
Cheers to making informed choices in AI and revolutionizing the way we interact with technology! 🚀 Until next time, keep exploring, learning, and innovating!
Keywords and related intents:
Keywords:
1. Artificial Intelligence
2. Generative AI
3. Discriminative AI
4. AI developer
5. Data generation
6. DALL-E
7. OpenAI
8. Classification tasks
9. DrawMyText
10. Text-to-image generation
11. Data augmentation
12. Predictive modeling
13. Email filtering
14. Disease diagnosis
15. Language translation
16. Computational resources
17. Technical decision
18. AI-driven artistry
19. Creativity
20. Innovation
Search Intents:
1. Difference between Generative AI and Discriminative AI
2. Applications of Generative AI in art and design
3. How to choose the right AI model for a specific task
4. Understanding the capabilities of DALL-E for image generation
5. Exploring DrawMyText pricing and features for AI-generated images
6. Generative AI’s role in creative tasks and data augmentation
7. Discriminative AI’s efficiency in classification and prediction tasks
8. Computational requirements for training Generative AI models
9. Examples of Discriminative AI in real-world applications like disease diagnosis
10. Overview and comparison of AI models in terms of complexity and data requirements
#generative ai vs. discriminative ai
#Choosing #Generative #Discriminative #Comprehensive #Guide