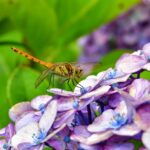
Choosing Between Generative AI and Discriminative AI: A Comprehensive Guide
January 11, 2024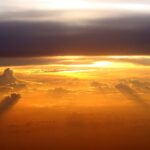
The Ultimate Guide to GPT-4: Unveiling Its Advanced Features and Capabilities
January 12, 2024Hello, AI enthusiasts and curious minds alike! I’m here to guide you through an exciting journey into the world of deep learning and its transformative impact on generative AI technologies. If you’ve heard the buzz but aren’t quite sure what all the fuss is about, fear not! We’re about to unravel the intricacies of this tech evolution together – and trust me, it’s fascinating stuff!
As we delve into the realm of artificial dreams and algorithms that mimic human creativity, we stand at the threshold of a new era. A time where our machines don’t just calculate; they create. Together, let’s explore how deep learning is breaking new ground in the field of generative AI and what it means for our future.
So grab a cup of your favorite beverage and settle in. It’s going to be an enlightening ride!
The Synergy of Deep Learning in Generative AI: An Overview
At its core, deep learning is a subset of machine learning that utilizes neural networks with many layers (hence the ‘deep’) to process data in complex ways. Think of it like an artisan refining their craft over time, each layer building upon the knowledge of the previous one to create a masterpiece. In the context of generative AI, this artisan becomes a creative force, generating content that is not only new but often startlingly human-like.
Generative AI refers to the process where AI systems create content, such as images, music, text, or even synthetic data sets. The role of deep learning in generative AI has been nothing short of revolutionary. Models like Generative Adversarial Networks (GANs) and transformer-based neural networks have given us glimpses of a world where AI-generated artwork sells for thousands and bots write convincing press releases.
The synergy of these technologies doesn’t just spell progress; it spells a seismic shift in how we conceive of creativity and the human/AI creative partnership. It’s an alliance that’s enhancing industries across the board, from fashion and film to pharmaceuticals and physics.
Illustrating Innovation: Deep Learning’s Impact on Creative AI Tools
Let’s zoom in on a few applications to see deep learning in generative AI at work. On the visual front, deep learning powers tools that generate realistic images and art from textual descriptions. This is not mere pixel pushing – it’s about creating visuals with an understanding of context, aesthetic, and even emotion.
For instance, our platform, DrawMyText, leverages such technologies to offer users a seamless experience in text-to-image generation. With intuitive tools and powerful deep learning algorithms, DrawMyText allows creators to bring their most imaginative concepts to life, all at the click of a button.
And it goes beyond static images. AI now animates scenes, fills gaps in old films, even designs virtual fashion for digital platforms. Each leap fueled by deep learning models that understand, interpret, and predict visual aesthetics and dynamics like never before.
Generative AI Technologies and Their Unseen Roles
While visual and content creation applications of generative AI are the most visible, deep learning’s tentacles reach into less visible, albeit equally transformative applications. Consider drug discovery, where AI models generate potential molecular structures for new pharmaceuticals, or climate models where they predict shifts in environmental patterns.
These applications may not make headlines with their generated content, but they represent deep learning in generative AI fulfilling critical roles that have the potential to save lives and protect our planet. They exemplify the depth and breadth of generative models’ capabilities, proving that what we see is only the tip of the iceberg.
The same models that dream up images for entertainment today could be the prototypes for systems that will generate life-saving innovations tomorrow. Such is the promise of this technology.
Embracing the Future: The Potential and Challenges Ahead
The role of deep learning in generative AI is undeniably significant, but it’s not without its challenges. Concerns around authenticity, ethical creation, and the potential for misuse must be tackled as we march towards a more AI-integrated future.
Moreover, the computing power required to train these sophisticated models is immense, leading to discussions around sustainability and accessibility. To democratize this technological revolution, efforts such as TensorFlow Lite are crucial, allowing for the deployment of AI models on mobile and edge devices.
Yet, despite these challenges, the potential for positive impact is monumental. As we cultivate AI that can create, the doors swing open to endless possibilities. From personal AI art assistants to automated R&D departments, the future looks bright – and incredibly inventive.
Conclusion: The Creative Convergence of AI and Humanity
As we wrap up our exploration into the revolutionary world of deep learning in generative AI, it’s clear that we stand on the cusp of a new creative zenith. A fusion of human ingenuity and algorithmic intelligence is enriching the tapestry of our world in ways previously unimaginable.
Whether it’s through giving life to the visions of artists with platforms like DrawMyText or accelerating scientific discoveries for the benefit of all, the impact of these technologies is profound. The key lies in harnessing them responsibly, ethically, and sustainably.
Thank you for joining me on this thrilling exploration of AI’s generative frontier. If you’re eager to be part of this creative revolution, consider subscribing to our premium text-to-image platform, DrawMyText. Together, let’s witness and shape the awe-inspiring future that generative AI promises. Stay curious, and let’s create wonders together! 😄
FAQ: Deep Learning and Generative AI Technologies
What is deep learning in the context of generative AI?
Deep learning refers to a class of machine learning that uses neural networks with many layers to learn from data. In generative AI, it helps create complex models capable of generating new, original content such as images, text, and music that are often indistinguishable from human-created content.
How do deep learning models learn to generate new content?
These models are trained on large datasets, learning patterns, styles, and structures. They then use this learned information to generate new content by sampling from the learned data distribution or by optimizing against a criteria defined by adversarial networks or other methods.
What are some popular deep learning architectures used in generative AI?
Generative Adversarial Networks (GANs), Variational Autoencoders (VAEs), and transformer-based models are among the most popular architectures. Each has its own mechanism for generating new content.
Are there any ethical concerns with generative AI?
Yes, ethical concerns include the potential for creating misleading or harmful content, issues surrounding the ownership of AI-generated content, and biases present in the training data being reflected in the generated content.
Can anyone use generative AI technology, and how accessible is it?
While advanced usage of generative AI may require technical expertise, platforms like DrawMyText make it accessible for non-technical users. Furthermore, open-source libraries and lightweight models are making these technologies more widely available.
Keywords and related intents:
Keywords:
1. AI enthusiasts
2. Deep learning
3. Generative AI
4. Creative technologies
5. Artificial creativity
6. Generative Adversarial Networks (GANs)
7. Transformer-based neural networks
8. Text-to-image generation
9. Ethics and authenticity
10. TensorFlow Lite
11. AI integration in industries
12. AI-powered drug discovery
13. Climate model prediction
14. Ethical AI creation
15. Accessibility of AI technologies
16. Deep learning model training
17. AI art tools
18. DrawMyText
19. AI animation
20. AI-generated content ownership
Search Intents:
1. Understand the basics of deep learning in AI.
2. Explore the impact of deep learning on creative AI applications.
3. Learn about the role of GANs in generative AI.
4. Find out how transformer neural networks contribute to AI advancements.
5. Discover platforms for text-to-image generation using AI.
6. Investigate the use of AI in animation and visual arts.
7. Understand the ethical considerations of generative AI.
8. Seek solutions for training deep learning models sustainably.
9. Find applications of AI in industries other than media, like pharmaceuticals.
10. Learn about making generative AI technologies more accessible and user-friendly.
#deep learning in generative ai
#Breaking #Ground #Role #Deep #Learning #Generative #Technologies